
International Research Journal of Finance and Economics, Vol. Long-term Dependencies and the Profitability of Technical Analysis.
A MULTIFRACTAL WALK DOWN WALL STREET SERIES
A Study on Spurious Long Memory in Nonlinear Time Series Models. An artificial neural network (p,d,q) model for timeseries forecasting. Financial Development and Economic Growth: A Review and New Evidence. Integration of nonlinear independent component analysis and support vector regression for stock price forecasting. Kao, L.J., Chiu, Ch.Ch., Lu, Ch.J., Yang, J.L. International Research Journal of Finance and Economics, 42, PP. Return and Volatility Contagions of Financial Markets over Difference Time Scales. Journal of International Financial Markets, Institutions and Money, Vol. Financial markets and economic growth in Greece, 1986–1999. Hondroyiannis, G., Lolos S., Papapetrou, E. International Journal of Forecasting, PP. Efficient market hypothesis and forecasting. An introduction to long memory time series models and fractional differencing. The Estimation and Application of Long-Memory Time Series Models. Recent Advances in Applied & Biomedical Informatics and Computational Engineering in Systems Applications, WSEAS Press, PP.412-417. Evidence of Improvement in Neural-Network Based Predictability of Stock Market Indexes through Co-movement Entries. International Journal of Machine Intelligence, ISSN: 0975–2927, Vol. Application of Artificial Neural Network for stock Market Predictions: A review of literature. Market Crash, Working Paper Kent State University, Department of Finance. Multifractal Spectral Analysis of the 1987 Stock. Forecasting Taiwan’s major stock indices by the Nash nonlinear grey Bernoulli model. The New Palgrave: A Dictionary of Economics, Vol.

Simple technical trading rules and the stochastic properties of stock returns. Working paper, University of Wisconsin at Madison, University of Houston, and University of Chicago, No. A Test of Independence Based on the Correlation Dimension. ěrock, W.A., Dechert, W.D., Sheinkman J.A. Long memory processes and fractional integration in econometrics. University of Pretoria Working Paper, No.
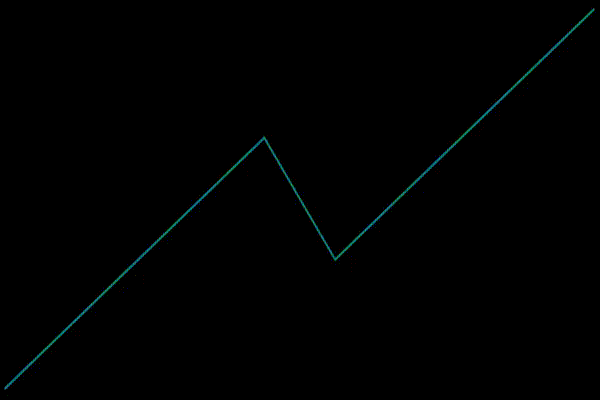
Predicting BRICS Stock Returns Using ARFIMA Models.

Ěye, G.C., Balcilar, M., Gupta, R., Kilimani, N., Nakumuryango, A., Redford, S. Effects of financial liberalization on financial market development and economic performance of the SSA region: An empirical assessment, Economic Modelling, Vol. G - Financial Economics > G1 - General Financial Markets > G10 - General Stock Return, Long Memory, NNAR, ARFIMA, Hybrid ModelsĬ - Mathematical and Quantitative Methods > C2 - Single Equation Models Single Variables > C22 - Time-Series Models Dynamic Quantile Regressions Dynamic Treatment Effect Models Diffusion ProcessesĬ - Mathematical and Quantitative Methods > C4 - Econometric and Statistical Methods: Special Topics > C45 - Neural Networks and Related TopicsĬ - Mathematical and Quantitative Methods > C5 - Econometric Modeling > C53 - Forecasting and Prediction Methods Simulation Methods Item Type:įinancial Time Series Forecasting by Developing a Hybrid Intelligent System The results further indicate that although dynamic artificial neural network model have a stronger performance compared to ARFIMA model, taking into consideration the inherent features of a market and combining it with neural network models can yield much better results. Our results showed that fractal markets hypothesis was confirmed in TSE therefore, it can be concluded that the fractal structure exists in the return of the TSE series. Finally, in order to introduce a complete design of a neural network for modeling and forecasting of stock return series, the long memory feature and dynamic neural network model were combined. In addition, fractal markets hypothesis investigates in daily data of the Tehran Stock Exchange (TSE) index. For this purpose, the present study tries to examine the capabilities of two sets of models, i.e., those based on artificial intelligence and regressive models. On this basis, in recent years, using intelligence-based techniques for forecasting has proved to be extremely successful and also is an appropriate choice as approximators to model and forecast time series, but designing a neural network model which provides a desirable forecasting is the main concern of researchers. The design of models for time series forecasting has found a solid foundation on statistics and mathematics.
